I am an assistant professor in the Materials Science and Engineering department at the University of Washington, specializing in advanced composite materials. My research team merges materials science, data science, and advanced manufacturing, focusing on three main areas: 1) Smart testing methods combining theory-guided machine learning and traditional techniques; 2) Smart manufacturing using automation, sensing, and machine learning; 3) Smart engineering methods to accelerate aerospace certification and qualification through machine learning and simulations. Our research is anchored in a novel machine learning framework that blends probabilistic and deterministic approaches, integrating engineering data and physical laws. This approach has led to several patented AI innovations for the aerospace sector.
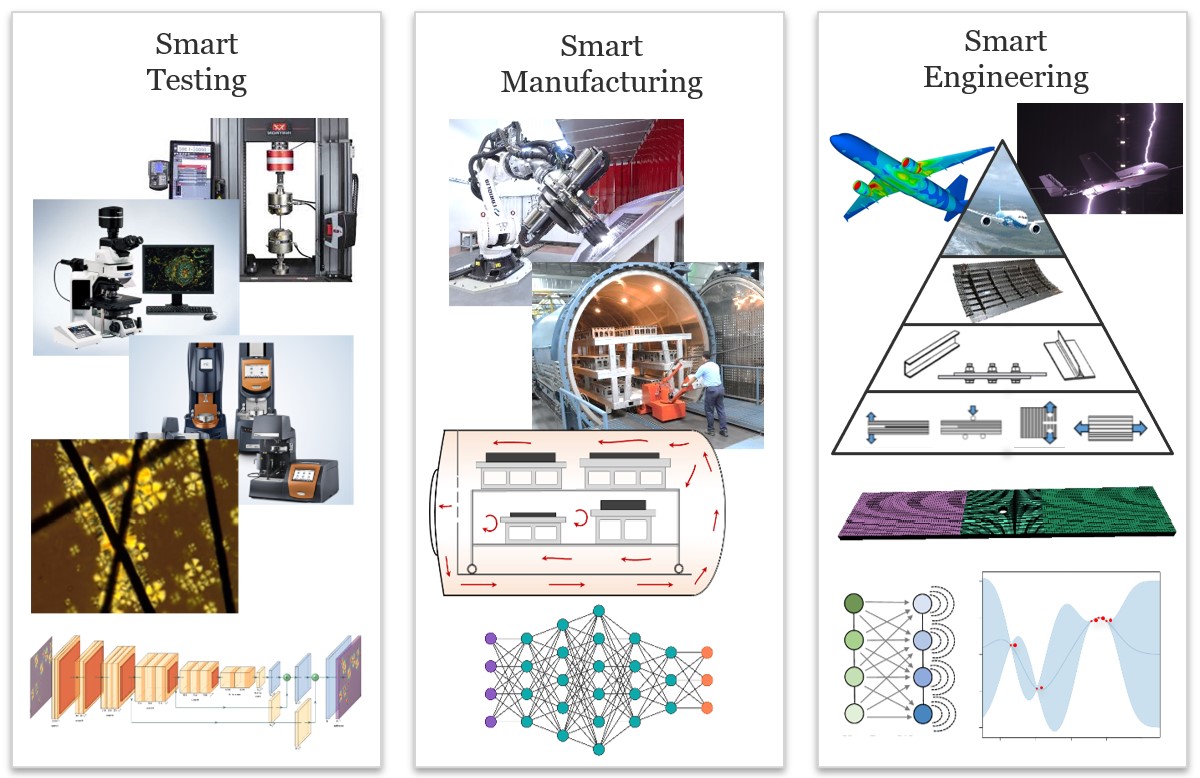
Recent Linkedin Posts
Recent Youtube Videos
Recent Awards
Online Courses
Selected Publications
Schoenholz, C., Li, S., Bainbridge, K., Huynh, V., Gray, A., & Zobeiry, N. (2023). Accelerated In Situ Inspection of Release Coating and Tool Surface Condition in Composites Manufacturing Using Global Mapping, Sparse Sensing, and Machine Learning. Journal of Manufacturing and Materials Processing, 7(3), 81. Lee, A., Wynn, M., Quigley, L., Salviato, M., & Zobeiry, N. (2022). Effect of temperature history during additive manufacturing on crystalline morphology of PEEK. Advances in Industrial and Manufacturing Engineering, 4, 100085. Humfeld, K. D., Gu, D., Butler, G. A., Nelson, K., & Zobeiry, N. (2021). A machine learning framework for real-time inverse modeling and multi-objective process optimization of composites for active manufacturing control. Composites Part B: Engineering, 223, 109150.